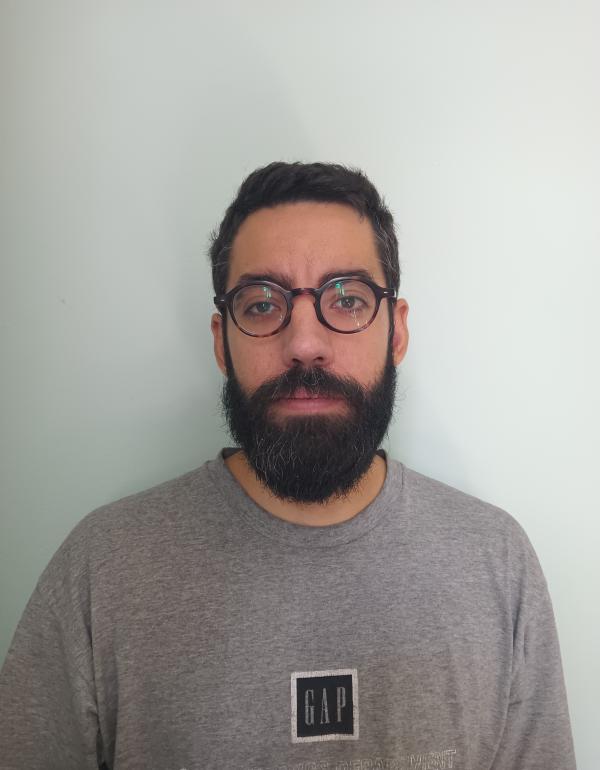
Periklis Tsiros received his diploma in Chemical Engineering from NTUA in 2017, and then obtained his MSc in Data Science at the department of Informatics in the Athens University of Economics and Business (AUEB ). He is currently pursuing a Ph.D. on stochastic modelling and control of systems in biosciences in the Unit of Process Control and Informatics at the School of Chemical Engineering, NTUA, where he is also employed as a junior researcher. His main professional interests consist of modelling of biological systems, Machine Learning, and Physiologically Based Pharmacokinetic modelling.
OpenTox 2022 Virtual Conference
A new method for grouping and estimating PBPK model parameters using genetic algorithms
Periklis Tsiros, Haralambos Sarimveis
School of Chemical Engineering, National Technical University, Athens, Greece
Physiologically Based PharmacoKinetic (PBPK) models are multicompartment models that can transform an external exposure into internal distribution by simulating complex time-varying kinetic processes. In mathematical terms, PBPK models are systems of differential equations, which express the time-dependent biouptake and biodistribution processes of chemical substances in humans and other animal species. One of the key strengths of these models is the mechanistic detail with which they describe the physiology of the body and the different tissues, which allows to perform species, gender, age or dose extrapolations. However, this level of detail involves a plethora of parameters that need to be estimated. Multiparametric PBPK models usually face identifiability and estimability issues, i.e. their parameters cannot be uniquely estimated, either due to lack of data or due to structural properties of the system of differential equations. Most model parameterisation methods which are in practice today, aim at estimating different values of each parameter across the various compartments or producing a single parameter value for all compartments. In this work, we present a novel approach that lies between the two extremes. Specifically, a genetic algorithm is designed with the aim of grouping the parameters across the various compartments, based on goodness-of-fit criteria. The method is demonstrated through a case study concerning the biodistribution of TiO2 nanoparticles after intravenous injection in rats. Different grouping strategies and goodness-of-fit criteria were tested. The results illustrated that by grouping the partition and permeability coefficients, we can produce a PBPK model with fewer parameters and better evaluation metrics compared to the conventional approaches. The results also highlighted that the concept of parameter grouping can support and improve the development of complex models in data-scarce environments.