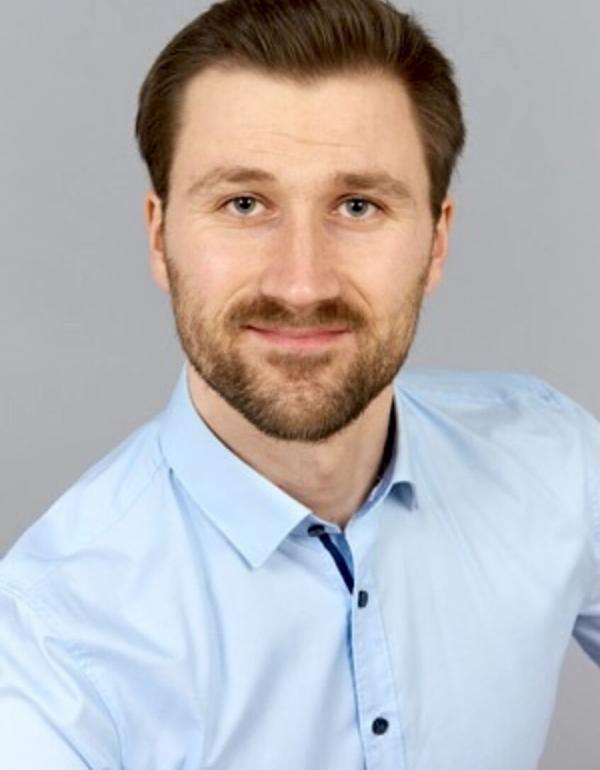
Palle Steen Helmke is a PhD student in the RISK-HUNT3R project, focusing on the development of KNIME workflows for predicting drug-induced liver injury (DILI), specifically cholestasis and steatosis. Publicly available target prediction tools such as Similarity Ensemble Approach (SEA), TargetNet, ChEMBL Conformal Prediction, and the ChEMBL Multitask Neural Network enrich the known compound-target interaction matrix through consensus scoring. These enriched data, along with compound/pathway interaction fingerprints and chemical fingerprints such as PubChem fingerprints, serve as input for machine learning models aimed at predicting toxicities. Modeling approaches like undersampling, consensus modeling, and probability range filtering are also applied to enhance model robustness and improve prediction accuracy. In a separate project, Palle explores bioisosterism, a key concept in medicinal chemistry involving the replacement of chemical groups to maintain biological activity. He developed a KNIME workflow that automates the generation and analysis of common bioisosteric replacements, focusing on esters, amides, carboxylic acids, and benzene rings within the ChEMBL database. The workflow retrieves pChEMBL values for 88 off targets, comparing the bioactivity of original compounds with their bioisosteric analogs, providing insights into how substitutions influence potency and toxicity. By combining cheminformatics, predictive modeling, and machine learning, Palle contributes to advancing computational toxicology and chemical safety assessment, bridging data science and medicinal chemistry to better understand and predict the biological and toxicological effects of chemical compounds.
OpenTox Summer School 2025
In Silico Target Prediction using KNIME
In silico target prediction is a computational method that identifies potential biological targets for compounds based on their chemical structures. This workshop will introduce participants to SEA (Similarity Ensemble Approach), a publicly available tool that predicts targets by comparing the chemical similarity of compounds to known compounds with established targets. The workshop will demonstrate how SEA can be integrated into KNIME, an open-source platform that enables users to design data science workflows without coding. Participants will gain practical, hands-on experience in using KNIME to expand the compound-target interaction matrix from ChEMBL by incorporating predicted interactions from SEA. This method enriches experimental data and provides a more comprehensive understanding of compound-target relationships, enhancing datasets for drug discovery and toxicology research.