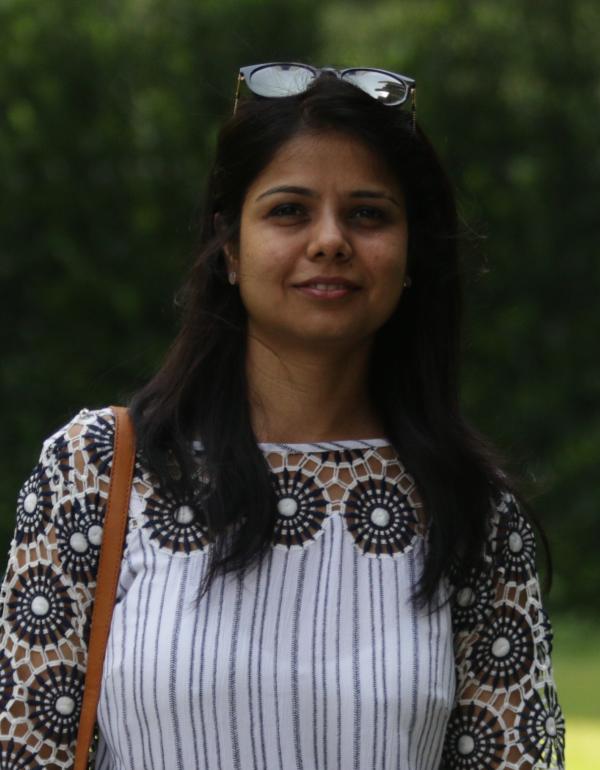
Dr Rama Kaalia is a Research Fellow at Biomedical Informatics Lab in Nanyang Technological University, Singapore. Dr Kaalia has a PhD from Jawaharlal Nehru University in Computational & Systems Biology where her work focused on understanding various aspects of disease-target-drug relationships. Main areas of research include in-silico toxicity prediction, ontology development, integration of heterogeneous biomedical data, network analysis and pharmacophore studies. Most of her PhD and post-doctoral research work has addressed need to analyse the integrated network of disease-target interactions to predict novel targets for a disease, identifying functional modules in proteome, pharmacophore based approach to design multi-target anti-malarials ab initio, and building predictive models for in silico toxicity prediction using chemical structure-function relationships.
Abstract OpenTox Asia 2019
Learning from chemical structures for in silico toxicity prediction
Rama Kaalia and Indira Ghosh
Toxicity causes failure in drug development at pre-clinical and clinal level. In silico drug toxicity prediction alleviates the high cost, long time and need of animal based experiments in drug discovery process. Chemical structures hold the key for information about toxicity effects of a drug. We present here an approach where a structural dictionary is prepared from experimentally known toxic and non-toxic compounds. The derived structural keys are then used to learn patterns responsible for toxicity. We demonstrate different machine learning based toxicity prediction models for different types of toxicity such as carcinogenicity and mutagenicity. Main focus of the presentation will be how machine learning methods such as support vector machines, random forests and deep learning can learn from structural alerts for predicting toxicity of the unknown compounds. Significant structural fragments responsible for toxicity of the chemicals are also calculated based on the best model leading in reducing toxicity from useable compounds.