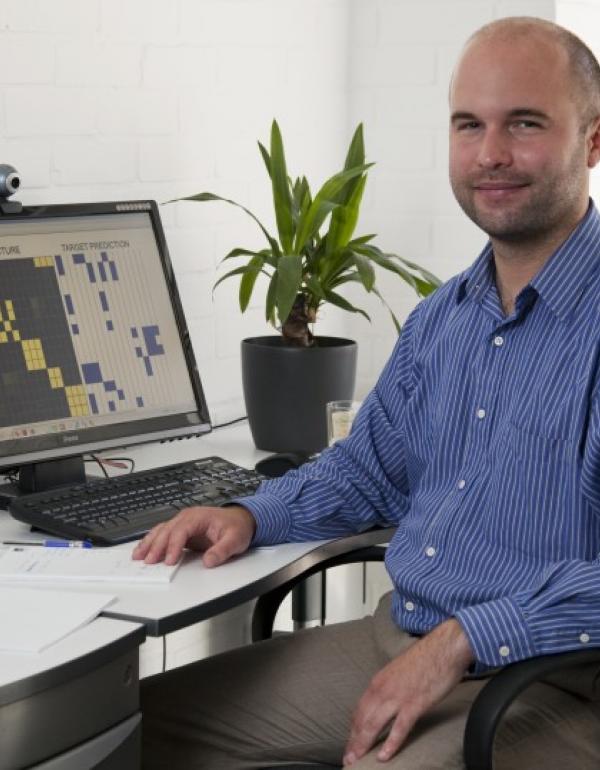
OpenTox Euro 2019 talk: Computational approaches for predicting compound safety and toxicity – Methods and applications
While the various mechanisms of potential compound toxicity are often difficult to model, and hence being confident about computational predictions can appear risky, we by now have significant amounts of chemical and biological data at our disposal that we can, and need to, put to good use in this domain[1].
In this presentation we will cover different methods and applications how computational approaches – based on existing experimental data and suitable modelling methods – can be used to predict compound safety or toxicity, and applied in a practical drug discovery setting.
These approaches will cover the utilization of gene expression data to guide compound selectivity during the lead optimization phase in a large pharmaceutical company[2], and also more generally discuss the use of ‘-omics’ data for anticipating compound toxicity[3]. Furthermore, we have been active in integrating additional types of information of chemical and biological nature for the prediction of compound toxicity, which in some cases was able to extend the applicability domain of predictive models for acute toxicity in rat[4].
Methodologically, given the limitations of the data we have, it is crucial to utilize modelling methods which are aware of the applicability domain of the models generated in the first place – Conformal Prediction is one such method which we have developed further and used in our group for cytotoxicity prediction in the recent past[5], which the modelling method being equally applicable to other types of toxicity data.
Finally, we have recently moved into using chemical and biological data from different domains to construct Adverse Outcome Pathways (AOPs) from data, such as for Structural Cardiotoxicity as an endpoint[6]. While data (and in particular data overlap between different readouts in compound structural space) remains a limiting factor, this should be improved by future studies which aim at utilizing data from historical drug discovery projects in pharmaceutical industry. In addition, the quantification of such pathways, and anticipating the likelihood of particular Molecular Initiating Events leading to particular Adverse Outcomes, will be an area of intense future research in the group.
References:
[1] The challenges involved in modeling toxicity data in silico: a review. MP Gleeson, S Modi, A Bender, RLM Robinson, J Kirchmair, M Promkatkaew, S Hannongbua, RC Glen – Current Pharmaceutical Design (2012) 18, 1266.
[2] Using transcriptomics to guide lead optimization in drug discovery projects: Lessons learned from the QSTAR project. B Verbist, G Klambauer, L Vervoort, W Talloen, Z Shkedy, O Thas, A Bender, HWH Göhlmann, S Hochreiter – Drug Discovery Today (2015) 20, 505.
[3] Developments in toxicogenomics: understanding and predicting compound-induced toxicity from gene expression data. B Alexander-Dann, LL Pruteanu, E Oerton, N Sharma, I Berindan-Neagoe, D Módos, A Bender – Molecular Omics (2018) 14, 218.
[4] Improving the prediction of organism-level toxicity through integration of chemical, protein target and cytotoxicity qHTS data. CHG Allen, A Koutsoukas, I Cortés-Ciriano, DS Murrell, TE Malliavin, RC Glen, A Bender – Toxicology Research (2016) 5, 883.
[5] Modelling compound cytotoxicity using conformal prediction and PubChem HTS data.F Svensson, U Norinder, A Bender – Toxicology Research (2017) 6, 73.
[6] Information-Derived Mechanistic Hypotheses for Structural Cardiotoxicity. F Svensson, A Zoufir, SY Mahmoud, AM Afzal, I Smit, KA Giblin, JT Mettetal, A Pointon, JS Harvey, N Greene, RV Williams, A Bender – Chemical Research in Toxicology (in preparation).