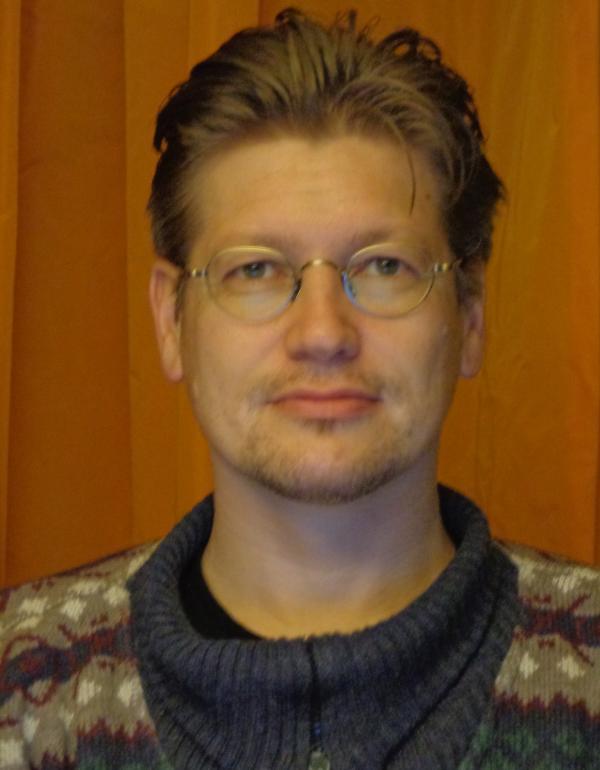
Dr. Danyel Jennen is Associate Professor in the field of toxicoinformatics at the Department of Toxicogenomics and he heads its core unit on toxicoinformatics. His research focuses on the development of complex bioinformatics models using a systems biology/toxicology approach and on constructing functional networks based on integrating multi-omics and phenotypic data. His work has been published in over 80 peer-reviewed publications (h-index 24). Within various multicenter (inter)national projects (from the Netherlands Toxicogenomics Centre, the EU FP6 & 7 Integrated Projects carcinoGENOMICS and diXa, and EU Horizon 2020 Project EU-ToxRisk) he was/is involved in the bioinformatics work on toxicogenomics in cancer hazard and (hepato)toxicity assessment. He recently coordinated the DECO2 project (Cefic-LRI AIMT4) on the use of non-animal data to supplement and strengthen read-across, commissioned by CEFIC, the European Chemical Industry Council. Furthermore, he was work package leader in the EU Horizon 2020 Project OpenRiskNet. Currently, he is work package leader in the national project BReIN on the development of an e-infrastructure for neurohealth and involved in the integrated data analyses of the Horizon 2020 IMI2 Project TransQST. Furthermore, he is a member of the HESI emerging Systems Toxicology for Assessment of Risk (eSTAR) Committee since October 2019.
Machine learning based meta-analysis using multiple toxicogenomics datasets does not improve genotoxicity prediction
Toxicogenomics-based approaches using in vitro cell models, developed as alternatives to animal testing, have been shown to be able to predict genotoxicity in vivo with accuracies above 85%, thereby outperforming the standard test batteries required by regulatory agencies. Despite their performance these approaches are not widely used yet due to the limited number of compounds used, the imbalance in classes, and/or the type of cell model used. In this study, we aim to overcome these hurdles by performing a meta-analysis on the large amounts of toxicogenomics data sets that have been generated the past decade, thereby further improving in vivo genotoxicity prediction. From the diXa Data Warehouse, ArrayExpress, GEO and Open TG-GATEs we collected gene expression data for human, rat and mouse in vitro liver cell models exposed to 156, 88 and 44 compounds with known genotoxicity information, respectively, at different time points and dosages resulting in 853, 702 and 100 experiments, respectively. The obtained datasets were merged and pre-processed per species. Species specific prediction models were built by using 10 machine learning algorithms on 10 train/test sets, each containing 80% of the data for training and 20% for testing. To avoid bias and possibly overfitting experiments using the same compound were all placed in either the training or test set. Support Vector Machines algorithm had the best accuracy for predicting genotoxicity in vivo at 69-82% with 81-93% specificity and 46-61% sensitivity. In conclusion, the meta-analysis did not improve in vivo genotoxicity prediction.