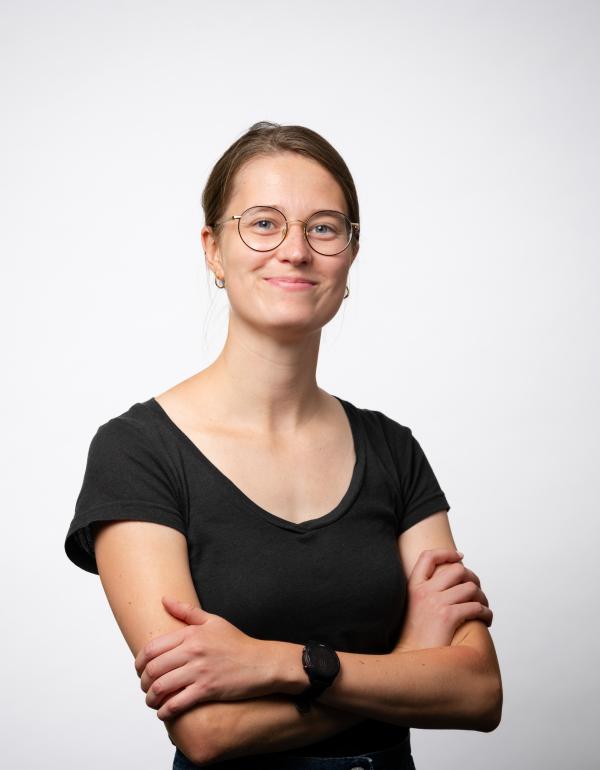
She obtained a Master of Science in Drug Development at the KU Leuven in Belgium. During her academic internship at the Department of Drug Delivery and Disposition at KU Leuven, she gained insight into the mechanisms underlying drug-induced liver toxicity and non-animal methods. She continued this interest in starting a PhD at the Vrije Universiteit Brussel, where she is focusing on the development of a human-based in vitro test battery for the prediction of chemical-induced liver steatosis within the ONTOX project.
An optimized adverse outcome pathway network for chemical-induced liver steatosis
Anouk Verhoeven1, Jonas van Ertvelde1, Joost Boeckmans1, Alexandra Gatzios1, Ramiro Jover2, Birgitte Lindeman3, Graciela Lopez soop3, Robim Marcelino Rodrigues1, Anna Rapisarda2, Julen Sanz-Serrano1, Marth Stinckens1, Sara Sepehri1, Mathieu Vinken1, Jian Jiang1* and Tamara Vanhaecke1*
1. Entity of In Vitro Toxicology and Dermato-Cosmetology, Department of Pharmaceutical and Pharmacological Sciences, Vrije Universiteit Brussel, Belgium
2. Joint Research Unit in Experimental Hepatology, University of Valencia, Health Research Institute Hospital La Fe & CIBER of Hepatic and Digestive Diseases, Spain
3. Department of Chemical Toxicology, Norwegian Institute of Public Health, Norway
* Equally contributing senior authors
The field of chemical toxicity testing is shifting to address the limitations of traditional in vivo studies. This transition focuses on implementing innovative non-animal methods to enhance predictability and provide deeper insights into toxicity mechanisms. Adverse Outcome Pathway (AOP) networks play a crucial role in structuring existing mechanistic knowledge related to toxicological processes. However, AOP networks are continuously evolving and need frequent updates to integrate new data. In addition, regulatory challenges remain, primarily due to concerns about the reliability of the information these networks provide.
This study introduces a Weight-of-Evidence (WoE) scoring method, aligning with the tailored Bradford Hill criteria, to quantitatively assess the confidence in key event relationships (KERs) within AOP networks. The existing AOP network was optimized with the latest scientific knowledge extracted from PubMed using the SysRev platform for artificial intelligence-based abstract inclusion and standardized data collection. The resulting optimized AOP network, constructed using Cytoscape, visually represents confidence levels through node size (key event, KE) and edge thickness (KERs).
The analysis of 173 research papers resulted in 100 unique KEs and 221 KERs among which 72 KEs and 170 KERs, respectively, have not been previously documented in the prior AOP network or AOP-wiki. Notably, modifications in de novo lipogenesis, fatty acid uptake and mitochondrial beta-oxidation, leading to lipid accumulation and liver steatosis, obtained the highest KER confidence scores.
In conclusion, this study delivers a generic methodology for developing and assessing AOP networks. The quantitative WoE scoring method facilitates in assessing the level of support for KERs within the optimized AOP network, providing valuable insights for its application in scientific research and regulatory settings.