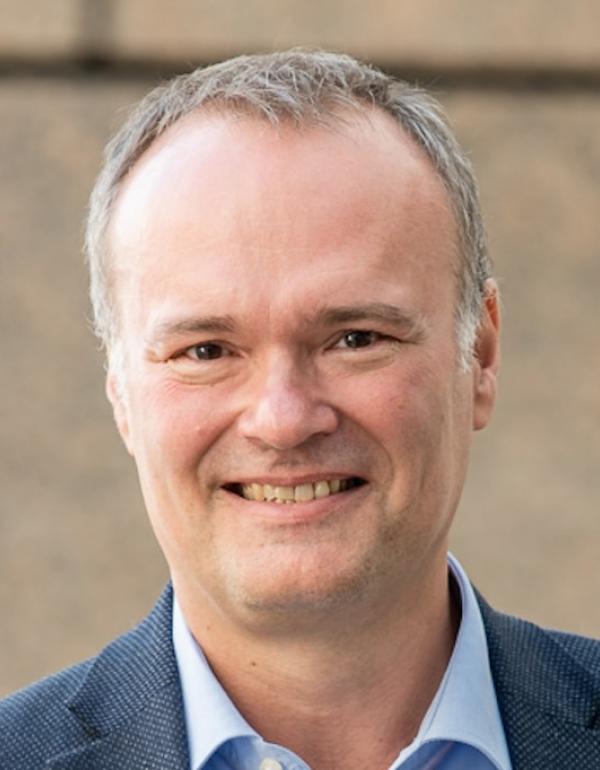
Gerhard Ecker is Professor of Pharmacoinformatics and Head of the Pharmacoinformatics Research Group at the Department of Pharmaceutical Sciences, University of Vienna. Gerhard received his doctorate in natural sciences from the University of Vienna and performed his post-doctoral training at the group of J. Seydel in Borstel (Germany). His research focuses on computational drug design with special emphasis on drug-transporter interaction and in silico safety assessment. He participated in 5 EU-funded projects related to toxicity prediction and animal free safety assessment and coordinated the Open PHACTS project, which created an Open Pharmacological Space by semantic integration of public databases. Gerhard served 2009 – 2011 as President of the European Federation for Medicinal Chemistry, and from 2018 – 2022 as Dean at the Faculty of Life Sciences.
In silico prediction of toxicity – let’s use fingerprints
Helmke P, Füzi B, Ecker, GF
University of Vienna, Department of Pharmaceutical Sciences, Vienna, Austria
The safety assessment of drugs is a critical factor of early development stages, necessitating rigorous testing to ensure efficacy and minimize harm to future patients. In recent years, new approach methods (NAMs) for animal free safety assessment have gained increasing importance, with in silico models becoming an integral part. A notable area of concern in drug safety is Drug-Induced Liver Injury (DILI), which remains a leading cause of drug withdrawal from the market, whereby cholestatic DILI constitutes 47 % of all drug-related liver injuries. As in vitro models for drug induced cholestasis (DIC) often focus on single parameters which may not fully capture its complexity, integrating in vitro with in silico approaches is essential.
However, due to the complexity of the endpoint, common molecular descriptors are not sufficient to fully capture all processes involved. Thus, recent generalized DILI models leverage substructure information, such as molecular descriptors (InterDILI), Graph Neural Networks (GeoDILI), and structural similarity-based DILI occurrence analysis (Toxstar). Füzi et al. built tree-based binary classification models utilizing targets and pathways, while Shin et al. have created a unified network that integrates relevant genes and pathways.
This study introduces a novel computational approach for modeling cholestasis, leveraging a panel of conceptionally diverse fingerprints. By integrating mechanistic and structural insights, our method enhances the explainability often missing in machine learning models used for toxicity prediction. Given the limited availability of experimental data on cholestasis in public databases, we additionally employed four publicly available target prediction tools to enrich our model. By employing an expanded consensus model of the top nine performing models and applying probability range filtering, the refined method achieved an MCC of 0.384 and a sensitivity of 0.802, thereby enhancing decision-making confidence. This approach advances DIC prediction by integrating biological and chemical fingerprints, offering a reliable and explainable model.
This work has received funding from the Austrian Science Fund (MolTag, grant W1232), and was supported by the project RISK-HUNT3R: RISK assessment of chemicals integrating HUman centric Next generation Testing strategies promoting the 3Rs. RISK-HUNT3R has received funding from the European Union's Horizon 2020 research and innovation programme under grant agreement No 964537 and is part of the ASPIS cluster. This work reflects only the authors’ views, and the European Commission is not responsible for any use that may be made of the information it contains.