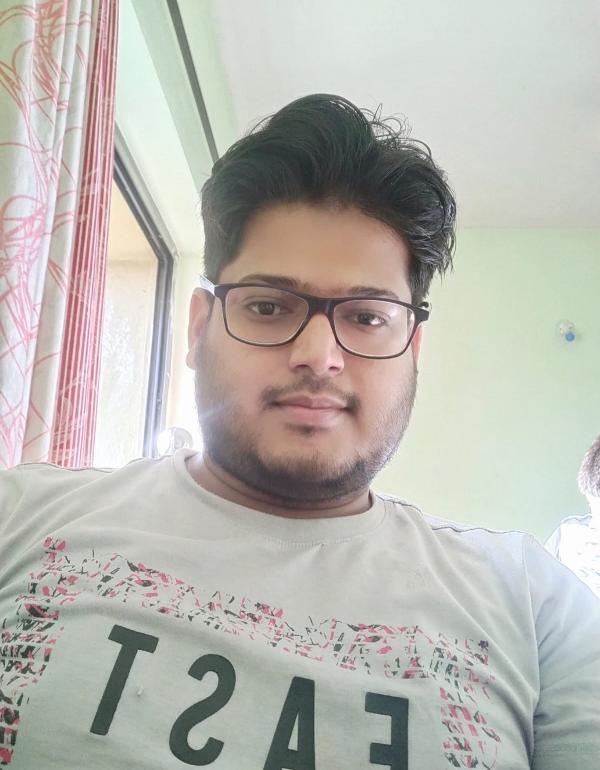
Dr. Kabiruddin Khan is an accomplished professional with over ten years of experience in plastic recycling, particularly through his roles as Project Manager and CEO at Faiz Traders in Goa, India. He has managed teams and overseen various recycling processes, including manual sorting, mechanical processing, and advanced methods like extrusion and injection molding to transform plastic waste into usable materials. Alongside his industry experience, Dr. Khan has been a core contributor to scientific projects across sectors, focusing on computational models for designing safer chemicals in fields ranging from pharmaceuticals to personal care and nanomaterials. His research has gained international recognition, amassing over 500 citations. Notably, his model on mutagenicity in nitro and amino aromatic compounds has been integrated into AlvaRunner, a software tool by AlvaScience in Italy. Dr. Khan also aims to launch a company focused on innovative environmental solutions. His vision encompasses tackling issues like chemical and plastic pollution and advancing waste management technologies to contribute to a sustainable future in the chemical industry.
A Predictive Framework for Cyanide Toxicity: Combining QSTR, SSD, and q-RASTR Approaches
Kabiruddin Khan1*, Ramin Abdullayev1#, Gopala Krishna Jillella2#, Varun Gopalakrishnan Nair1#, Mahmoud Bousily1, Supratik Kar3, Agnieszka Gajewicz-Skretna1*
1Laboratory of Environmental Chemoinformatics, Faculty of Chemistry, University of Gdansk, Wita Stwosza 63, 80-308 Gdansk, Poland.
2Department of Pharmaceutical Chemistry, Dr. K. V. Subba Reddy Institute of Pharmacy, Dupadu, Kurnool, Andhra Pradesh, India-518218.
3Chemometrics and Molecular Modeling Laboratory, Department of Chemistry and Physics, Kean University, 1000 Morris Avenue, Union, NJ 07083, USA
#Equal contribution as second authors.
*Corresponding Authors
Cyanide compounds, widely used in sectors such as mining, metallurgy, and chemical synthesis, present substantial environmental and health risks due to their toxicity. This study employs advanced predictive modeling techniques—Quantitative Structure-Toxicity Relationship (QSTR), Species cyanide-Sensitivity Distribution (ScSD), and quantitative Read
Across Structure Toxicity (q-RASTR)—to assess cyanide toxicity comprehensively. Using a dataset of 25 cyanide salts, the models predict acute, chronic, and lethal toxicity across species including humans, rats, and fish. Key molecular descriptors such as refractivity, solubility, and lipophilicity were calculated using ALOGPS 2.1, ChemAxon, and Elemental-Descriptor 1.0. The study develops machine learning models via Multiple Linear Regression (MLR), Partial Least Squares (PLS), and k-Nearest Neighbors (kNN), yielding predictive models validated with high accuracy. Furthermore, q-RASTR models utilize structural similarity to enhance predictive power by integrating features from QSTR and ScSD models. These combined approaches provide a robust framework for species-specific toxicity prediction, elucidating cyanide's molecular toxicity mechanisms and supporting regulatory risk assessments. The results are available for public access at https://cynaide-ml.onrender.com/.
Acknowledgment
This research is part of the project No. 2022/45/P/NZ7/03391 co-funded by the National Science Centre and the European Union’s Horizon 2020 research and innovation programme under the Marie Skłodowska-Curie grant agreement no. 945339. For the purpose of Open Access, the author has applied a CC-BY public copyright licence to any Author Accepted Manuscript (AAM) version arising from this submission.