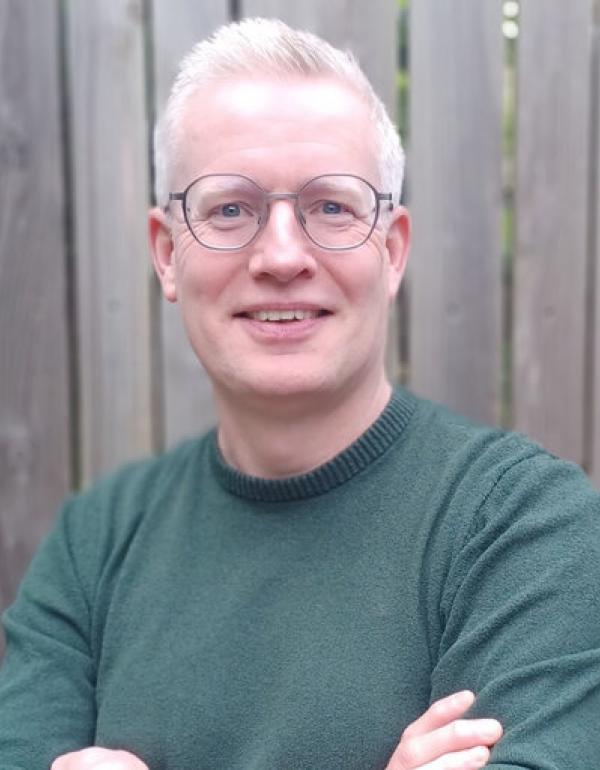
Marc has a wide range of interests. For the past 15 years, Marc has been involved in research to new approach methodologies (NAMs). He conducted research on the development and validation of methods for skin-sensitization, mutagenicity, developmental toxicity, and metabolism. Marc moved his attention in research from the wet lab to in-silico modelling and has become a proficient programmer and machine learning model developer. His current research focusses on the application of Artificial Intelligence in chemical risk assessment; the development and application of Large Language Models for synthesis of evidence and data extraction; and the implementation of open science and reproducible research principles in the toxicological research field.
For the development of the data science competences of the university, Marc is a driving force behind the formation of an organization wide data science group, that is intended to support many research projects in the organization. Furthermore, Marc frequently teaches data science workshops and trainings for postdoctoral researchers within the university and in large research collaborations such as ONTOX. In his capacity as data scientist, Marc regularly provides statistical advice and develops visualizations and interactive data exploration tools.
AI-facilitated workflows in ONTOX
Marie Corradi1,*, Devon Barnes4, Thomas Luechtefeld2,3, Jesse Egmond1, Mathieu Vinken5, Thomas Hartung3, Marc Teunis1#
1Innovative Testing in Life Sciences and Chemistry, Utrecht University of Applied Sciences, Utrecht, Netherlands
2ToxTrack, Bethesda, MD, United States
3Environmental Health and Engineering, Johns Hopkins Bloomberg School of Public Health, Baltimore, MD, United States
4 University Medical Center Utrecht
5Department of Pharmaceutical and Pharmacological Sciences, Vrije Universiteit Brussel-Belgium, Brussels, Belgium
#Presenting author
To study the ways in which compounds can induce adverse effects, toxicologists have been constructing Adverse Outcome Pathways (AOPs). An AOP can be considered as a pragmatic tool to capture and visualize mechanisms underlying different types of toxicity inflicted by any kind of stressor, and describes the interactions between key entities that lead to the adverse outcome on multiple biological levels of organization. The construction or optimization of an AOP is a labor intensive process, which currently depends on the manual search, collection, reviewing and synthesis of available scientific literature.
In this presentation, we will demonstrate ways to leverage AI - in particular language models – to augment the process of knowledge extraction and synthesis for AOPs and toxicology in general. We will showcase three examples on how natural language processing was used in toxicological data extraction workflows:
1. To reconstruct elements of a AOPs for liver toxicity, in particular cholestasis and steatosis
2. To extract relationships between biomedical entities, specifically in the cases of liver toxicity and Parkinson’s disease.
3. To facilitate evidence review to build an AOP for Kidney toxicity
We will provide an outlook on where the field of AI is heading with respect to knowledge extraction and synthesis, especially in the context of large language models, retrieval augmented generation (RAG) and knowledge graphs. Latest developments in the field such as agentic AI and graph-based RAG will be put in the context of the case examples and we will propose a future perspective on the implementation of these tools in the chemical hazard and risk-assessment framework.