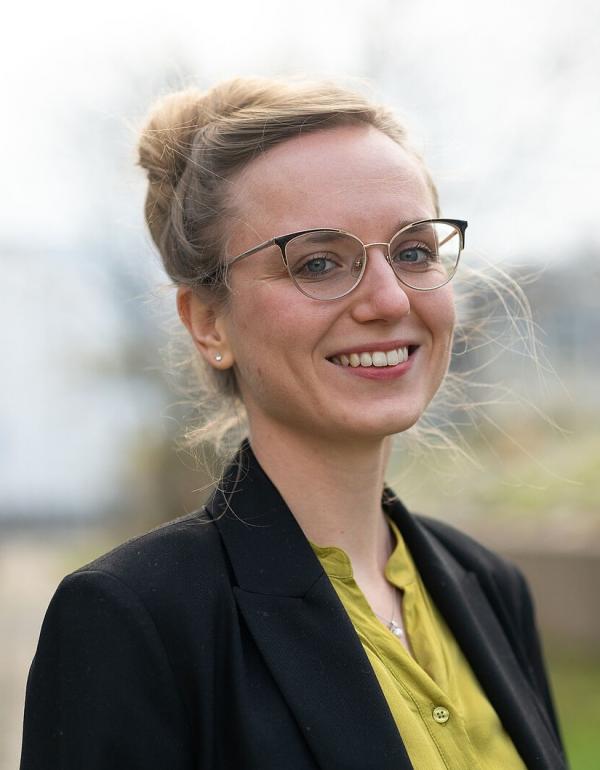
Roxane Jacob is a second-year PhD student in the Computational Drug Discovery and Design (COMP3D) group led by Assoc-Prof. Johannes Kirchmair at the University of Vienna. She is affiliated with the Christian Doppler Laboratory for Molecular Informatics in the Biosciences. Roxane holds master’s degrees in Organic Chemistry from the University of Freiburg and in Computational Science from the University of Vienna. Her research focuses on developing innovative in silico methods to understand and predict xenobiotic metabolism, with particular interests in geometric deep learning and uncertainty estimation.
AWESOM: a GNN-based Site-Of-Metabolism Predictor with Aleatoric and Epistemic Uncertainty Estimation
Roxane A. Jacob1,2,3, Oliver Wieder1,2, Ya Chen1, Angelica Mazzolari4, Andreas Bergner5, Klaus-Juergen Schleifer6, Johannes Kirchmair1,2,∗
1 Department of Pharmaceutical Sciences, Division of Pharmaceutical Chemistry,
Faculty of Life Sciences, University of Vienna, Josef-Holaubek-Platz 2, 1090 Vienna, Austria.
2 Christian Doppler Laboratory for Molecular Informatics in the Biosciences, Department for
Pharmaceutical Sciences, University of Vienna, Josef-Holaubek-Platz 2, 1090 Vienna, Austria
3 Vienna Doctoral School of Pharmaceutical, Nutritional and Sport Sciences (PhaNuSpo),
University of Vienna, Josef-Holaubek-Platz 2, 1090 Vienna, Austria
4 Dipartimento di Scienze Farmaceutiche, Universita degli Studi di Milano, I-20133 Milano, Italy
5 Drug Discovery Sciences, Boehringer Ingelheim RCV GmbH & Co KG, Vienna, Austria
6 BASF SE, 67063 Ludwigshafen am Rhein, German
Determining the metabolic fate of xenobiotics is essential to ensuring their safety and effectiveness. While in vivo and in vitro methods remain the golden standard for ascertaining the metabolic stability of novel compounds, they are both resource-intensive and time-consuming. In silico metabolism prediction models offer a complementary approach to enhance metabolic stability assessments while preserving desired biological activity. We present AWESOM, a graph neural network (GNN)-based site of-metabolism (SOM) predictor covering both phase 1 and 2 metabolic reactions. AWESOM utilizes deep ensemble learning to partition predictive uncertainty into aleatoric and epistemic components. We demonstrate how these uncertainty estimates increase prediction accuracy, provide actionable insights for model enhancement and data expansion, and streamline the process of experimental metabolic characterization for new compounds.