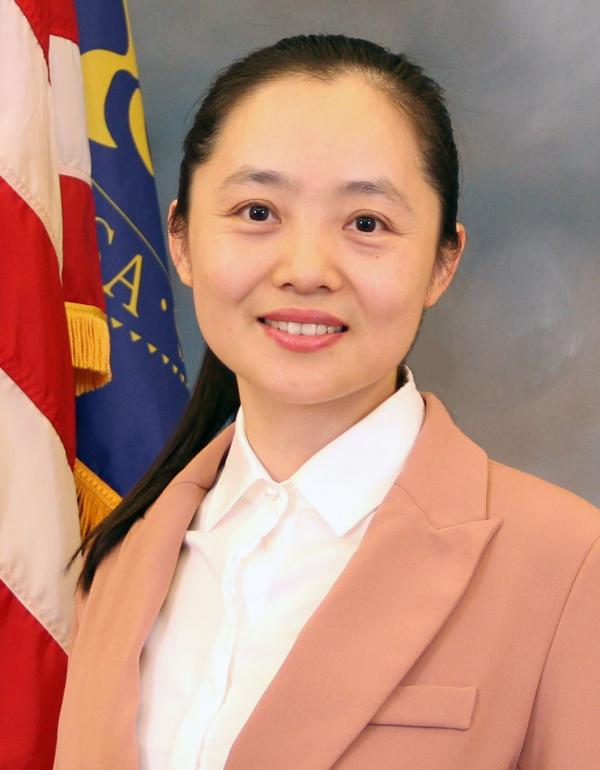
Dr. Ting Li received a Ph.D. in bioinformatics from the joint program at the University of Arkansas at Little Rock and the University of Arkansas for Medical Sciences. She then joined the Division of Bioinformatics and Biostatistics (DBB) at FDA’s National Center for Toxicological Research (NCTR) as an Oak Ridge Institute for Science and Education fellow. She was then converted to a visiting scientist with DBB. Throughout her career, Dr. Li has authored or co-authored more than 15 peer-reviewed publications and presented at nearly 20 academic conferences. In 2022, she was honored with the 1st place award in the Environmental Mutagenesis and Genomics Society's Bioinformatics Challenge.
Research Interests
Dr. Li’s research interests focus on developing and applying advanced artificial intelligence (AI) methodologies for drug safety research and the extension of their potential application in the FDA review process. This research includes:
• Developing quantitative structure-activity relationships (QSARs) models using advanced AI methodologies for various safety endpoints important to the FDA, such as drug-induced liver injury (DILI), carcinogenicity, and mutagenicity.
• Developing AI and machine learning models using genomic data to predict toxicological endpoints.
• Developing generative adversarial networks methods to translate genomic profiles from one domain to another, which aims to addressing data scarcity while enriching the analysis.
Session: AI in Risk Assessment Session
Chair: Ting Li, Ph.D., FDA/NCTR
Artificial Intelligence (AI) is reshaping risk assessment frameworks, particularly in toxicology, by tackling the growing complexity and volume of data. This session will explore how AI can understand of drug induced toxicity and gene expression changes, facilitating more efficient and accurate assessments. By showcasing advanced AI applications—such as AI-driven toxicity labeling, single-cell dose-response predictions, and regulatory insights—this session will underscore the transformative potential of AI in advancing regulatory science and enhancing public health protection. Bringing together experts from academia and regulatory bodies, this session aims to foster collaborative discussions on the seamless integration of AI into risk assessment frameworks, driving improvements in drug safety evaluation, regulatory decision-making, and public health.