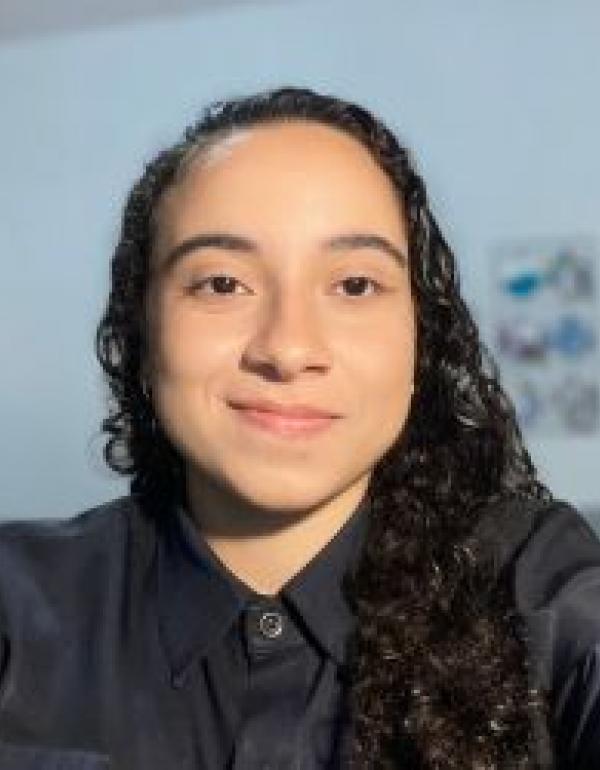
Ester Souza is an undergraduate student in Biotechnology at the Federal University of Goiás (UFG). She is a member of the Laboratory of Molecular Modeling and Drug Design (LabMol) and has been actively involved in research focusing on Artificial Intelligence and chemoinformatics. She also works as a junior developer and researcher at the Center of Excellence in Artificial Intelligence. Her most recent research is related to the application of Machine Learning and Deep Learning for the investigation of Chemical Endocrine Disruptors. She also develops research involving the development of AI models for predicting the toxicity of mixtures in aquatic environments. Ester has dedicated herself to the development of scientific tools that aim to improve the assessment of toxicity of chemical compounds and contribute to the area of computational toxicology.
Advanced Artificial Intelligence Models for the Prediction of Progesterone Endocrine Disruptors
Ester Souza, 1-3; Igor H. Sanches, 1-3and Carolina H. Andrade 1-3
1 Laboratory for Molecular Modeling and Drug Design (LabMol), Faculty of Pharmacy, Universidade Federal de Goiás, Goiânia, GO, Brazil.
2 Center for Excellence in Artificial Intelligence (CEIA), Institute of Informatics, Universidade Federal de Goiás, Goiânia, GO, Brazil.
3 Center for the Research and Advancement in Fragments and Molecular Targets (CRAFT), School of Pharmaceutical Sciences at Ribeirão Preto, University of São Paulo, Ribeirão Preto, SP, Brazil.
Presenting author: Ester Souza
The progesterone receptor (PR) regulates fundamental physiological processes such as development, metabolic homeostasis, reproduction, all driven by the progesterone. Exposure to endocrine disruptors (EDCs) can alter its function, being associated with health issues such as obesity, diabetes, and cancer. EDCs are exogenous compounds that interfere with hormones, mimicking or inhibiting their functions. In this study, we aimed to develop predictive artificial intelligence (AI) models to identify EDCs that affect the PR. Using the quantitative structure activity relationship (QSAR) approach, we linked chemical structures of compounds with their biological activity, enabling the prediction of untested compounds. The methodology included comprehensive data collection, curation, and standardization focused on known compounds that interact with the PR. After data standardization, chemical space analysis was performed using t-distributed Stochastic Neighbor Embedding (t-SNE), integrated with Natural Language Processing (NLP) to extract meaningful activity patterns from the dataset. The resulting AI models demonstrated strong performance metrics: for agonist compounds, the models achieved a balanced accuracy (BACC) of 0.82, sensitivity (Se) of 0.79, specificity (Sp) of 0.83, and a Matthew correlation coefficient (MCC) of 0.56. For antagonist compounds, the models exhibited a BACC of 0.81, Se of 0.80, Sp of 0.82, and MCC of 0.57. These results indicate the robustness of the models in distinguishing between active and inactive compounds with respect to PR. The models are currently being optimized and will soon be accessible through the InsightAI platform (http://insightai.labmol.com.br), providing a user-friendly tool for researchers and industry professionals to screen chemicals for their potential interference with progesterone hormonal action. This project also aligns with the “3Rs” (reduction, refinement, and replacement) principles in animal testing, demonstrating the potential of AI to minimize reliance on animal experimentation by providing reliable in silico alternatives. Integrating AI technologies with chemical data generates valuable insights to understand and mitigate risks associated with EDC exposure, ultimately benefiting public health and environmental safety.