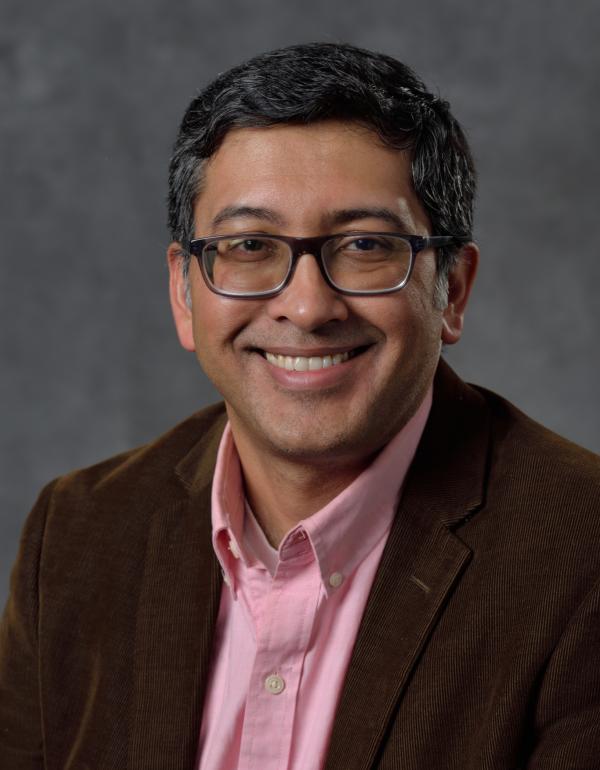
Sudin Bhattacharya is an Associate Professor in the Departments of Biomedical Engineering and Pharmacology & Toxicology, and the Institute for Quantitative Health Science and Engineering, at Michigan State University. He received his PhD in Mechanical Engineering from the University of Michigan in 2006, and did his post-doctoral training in Computational Toxicology at The Hamner Institutes for Health Sciences in North Carolina, working on deterministic and stochastic computational models of transcriptional networks underlying cellular differentiation and its perturbation by toxicants like dioxins. Dr. Bhattacharya started his own lab at Michigan State University in 2017, and earned tenure in 2023. He conducts research at the interface of computation and biology, using quantitative tools to study the signaling and transcriptional networks that regulate cell fate, and the perturbation of these networks by environmental pollutants. Dr. Bhattacharya is a recipient of the Young Investigator Award from the Association of Scientists of Indian Origin of the Society of Toxicology, and the Edward Carney Award for Predictive Toxicology from the American Society for Cellular and Computational Toxicology. His research has been supported by the US Environmental Protection Agency and the US National Institutes of Health.
Deep Generative Learning of Single Cell Gene Expression to predict Dose-Dependent Chemical Perturbations
Sudin Bhattacharya, PhD
Department of Biomedical Engineering, Department of Pharmacology and Toxicology Institute for Quantitative Health Science & Engineering, Michigan State University
Single cell RNA-sequencing allows us to study cell-type specificity and heterogeneity of biological responses to chemical perturbations. However, experimentally testing all relevant combinations of cell types, chemicals, and doses is a near-impossible task. A deep learning formalism called variational autoencoders (VAEs) has been shown to be effective in computationally predicting single-cell gene expression perturbations. We have developed single cell Variational Inference of Dose-Response (scVIDR), a VAE-based tool to predict the trajectory of cellular dose-response, which achieves better dose-response predictions than existing models. We show that scVIDR can predict dose-dependent gene expression changes across cell types and interpret the latent space of the autoencoder model in terms of biological pathways.